Releasing all datasets involved in the development of a Foundation Model, including training, fine-tuning, and evaluation data, can facilitate external scrutiny and support further research. Proper data governance practices, including respecting opt-out preference signals, pseudonymization, or PII redaction, are required at the curation and release stages. Data access control based on research needs and enabling data subjects to request removal from the hosted dataset are essential.
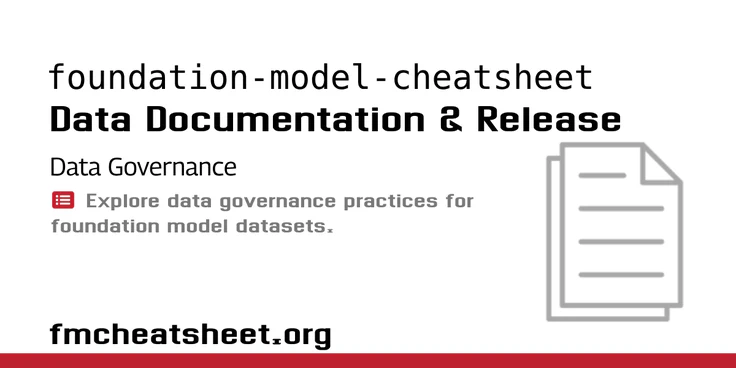