Taxonomies provide a way of categorising, defining and understanding risks and hazards created through the use and deployment of AI systems. The following taxonomies focus on the types of interactions and uses that create a risk of harm as well as the negative effects that they lead to.
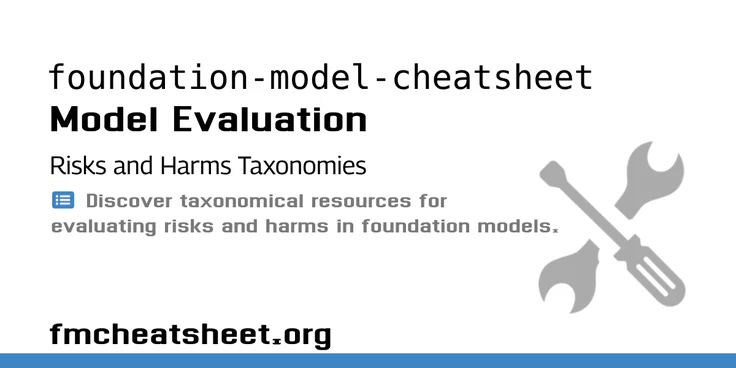